Qinghao Hu, Nanyang Technological University; Harsha Nori, Microsoft; Peng Sun, SenseTime; Yonggang Wen and Tianwei Zhang, Nanyang Technological University
While machine learning has demonstrated remarkable performance in various computer systems, some substantial flaws can prohibit its deployment in practice, including opaque decision processes, poor generalization and robustness, as well as exorbitant training and inference overhead. Motivated by these deficiencies, we introduce Primo, a unified framework for developers to design practical learning-augmented systems. Specifically, (1) Primo provides two interpretable models (PrAM and PrDT), as well as a Distill Engine, to support different system scenarios and deployment requirements. (2) It adopts Bayes Optimization to automatically identify the optimal model pruning strategy and hyperparameter configuration. (3) It also implements two tools, Monotonic Constraint and Counterfactual Explanation, to achieve transparent debugging and guided model adjustment. Primo can be applied to different types of learning-augmented systems. Evaluations on three state-of-the-art systems show that Primo can provide clear model interpretations, better system performance, and lower deployment costs.
Open Access Media
USENIX is committed to Open Access to the research presented at our events. Papers and proceedings are freely available to everyone once the event begins. Any video, audio, and/or slides that are posted after the event are also free and open to everyone. Support USENIX and our commitment to Open Access.

author = {Qinghao Hu and Harsha Nori and Peng Sun and Yonggang Wen and Tianwei Zhang},
title = {Primo: Practical {Learning-Augmented} Systems with Interpretable Models},
booktitle = {2022 USENIX Annual Technical Conference (USENIX ATC 22)},
year = {2022},
isbn = {978-1-939133-29-63},
address = {Carlsbad, CA},
pages = {519--538},
url = {https://www.usenix.org/conference/atc22/presentation/hu},
publisher = {USENIX Association},
month = jul
}
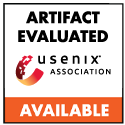
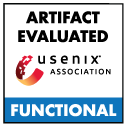
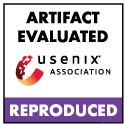