Haoran Qiu, Weichao Mao, Archit Patke, and Shengkun Cui, University of Illinois Urbana-Champaign; Saurabh Jha, Chen Wang, and Hubertus Franke, IBM Research; Zbigniew Kalbarczyk, Tamer Başar, and Ravishankar K. Iyer, University of Illinois Urbana-Champaign
With the increasing popularity of large deep learning model-serving workloads, there is a pressing need to reduce the energy consumption of a model-serving cluster while maintaining satisfied throughput or model-serving latency requirements. Model multiplexing approaches such as model parallelism, model placement, replication, and batching aim to optimize the model-serving performance. However, they fall short of leveraging the GPU frequency scaling opportunity for power saving. In this paper, we demonstrate (1) the benefits of GPU frequency scaling in power saving for model serving; and (2) the necessity for co-design and optimization of fine-grained model multiplexing and GPU frequency scaling. We explore the co-design space and present a novel power-aware model-serving system, µ-Serve. µ-Serve is a model-serving framework that optimizes the power consumption and model serving latency/throughput of serving multiple ML models efficiently in a homogeneous GPU cluster. Evaluation results on production workloads show that µ-Serve achieves 1.2–2.6× power saving by dynamic GPU frequency scaling (up to 61% reduction) without SLO attainment violations.
USENIX ATC '24 Open Access Sponsored by
King Abdullah University of Science and Technology (KAUST)
Open Access Media
USENIX is committed to Open Access to the research presented at our events. Papers and proceedings are freely available to everyone once the event begins. Any video, audio, and/or slides that are posted after the event are also free and open to everyone. Support USENIX and our commitment to Open Access.

author = {Haoran Qiu and Weichao Mao and Archit Patke and Shengkun Cui and Saurabh Jha and Chen Wang and Hubertus Franke and Zbigniew Kalbarczyk and Tamer Ba{\c s}ar and Ravishankar K. Iyer},
title = {Power-aware Deep Learning Model Serving with {μ-Serve}},
booktitle = {2024 USENIX Annual Technical Conference (USENIX ATC 24)},
year = {2024},
isbn = {978-1-939133-41-0},
address = {Santa Clara, CA},
pages = {75--93},
url = {https://www.usenix.org/conference/atc24/presentation/qiu},
publisher = {USENIX Association},
month = jul
}
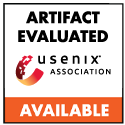
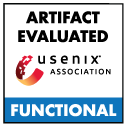
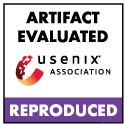