Hyrum Anderson, Microsoft
Researchers in adversarial machine learning (ML)—which studies the security of ML in the presence of an attacker—have written more than 2,300 papers in just the last 6 years. However, in a recent survey, almost 90% of corporations and government entities reported that they are not equipped to protect their ML models. The fact remains that there are significant gaps between academic advancements and industry needs and practices. First, aside from full-scale security products, ML models get scant security consideration. Second, most organizations who are concerned with ML security do not know how to begin. Third, the tools and techniques produced in academic research often don't translate to business needs.
To demonstrate these gaps, I will review some sobering lessons learned during a Machine Learning Red Team engagement at Microsoft on systems that rely on ML. They include the reality that "traditional" security measures like access control and permissions remain the most significant element for safeguarding ML systems. Furthermore, especially outside of security applications, awareness about ML vulnerabilities remains low and security posture is nearly zero.
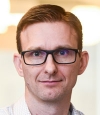
Hyrum Anderson is Principal Architect in the Azure Trustworthy Machine Learning group at Microsoft. Prior to joining Microsoft, he was the Chief Scientist at Endgame, and conducted research in information security and situational awareness at FireEye, Mandiant, Sandia National Laboratories, and MIT Lincoln Laboratory. He received his Ph.D. in Electrical Engineering (signal processing + machine learning) from the University of Washington and BS+MS degrees from Brigham Young University. He is co-founder and co-chair for the Conference on Applied Machine Learning in Information Security and has spoken at numerous signal processing, machine learning, and security conferences, including RSA, DEFCon, and BlackHat.
Open Access Media
USENIX is committed to Open Access to the research presented at our events. Papers and proceedings are freely available to everyone once the event begins. Any video, audio, and/or slides that are posted after the event are also free and open to everyone. Support USENIX and our commitment to Open Access.

author = {Hyrum Anderson},
title = {The Practical Divide between Adversarial {ML} Research and Security Practice: A Red Team Perspective},
year = {2021},
publisher = {USENIX Association},
month = feb
}