Zhuohan Li and Lianmin Zheng, UC Berkeley; Yinmin Zhong, Peking University; Vincent Liu, University of Pennsylvania; Ying Sheng, Stanford University; Xin Jin, Peking University; Yanping Huang and Zhifeng Chen, Google; Hao Zhang, UC San Diego; Joseph E. Gonzalez and Ion Stoica, UC Berkeley
Model parallelism is conventionally viewed as a method to scale a single large deep learning model beyond the memory limits of a single device. In this paper, we demonstrate that model parallelism can be additionally used for the statistical multiplexing of multiple devices when serving multiple models, even when a single model can fit into a single device. Our work reveals a fundamental trade-off between the overhead introduced by model parallelism and the opportunity to exploit statistical multiplexing to reduce serving latency in the presence of bursty workloads. We explore the new trade-off space and present a novel serving system, AlpaServe, that determines an efficient strategy for placing and parallelizing collections of large deep learning models across a distributed cluster. Evaluation results on production workloads show that AlpaServe can process requests at up to 10× higher rates or 6× more burstiness while staying within latency constraints for more than 99% of requests.
OSDI '23 Open Access Sponsored by
King Abdullah University of Science and Technology (KAUST)
Open Access Media
USENIX is committed to Open Access to the research presented at our events. Papers and proceedings are freely available to everyone once the event begins. Any video, audio, and/or slides that are posted after the event are also free and open to everyone. Support USENIX and our commitment to Open Access.

author = {Zhuohan Li and Lianmin Zheng and Yinmin Zhong and Vincent Liu and Ying Sheng and Xin Jin and Yanping Huang and Zhifeng Chen and Hao Zhang and Joseph E. Gonzalez and Ion Stoica},
title = {{AlpaServe}: Statistical Multiplexing with Model Parallelism for Deep Learning Serving},
booktitle = {17th USENIX Symposium on Operating Systems Design and Implementation (OSDI 23)},
year = {2023},
isbn = {978-1-939133-34-2},
address = {Boston, MA},
pages = {663--679},
url = {https://www.usenix.org/conference/osdi23/presentation/li-zhouhan},
publisher = {USENIX Association},
month = jul
}
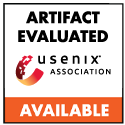
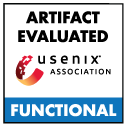
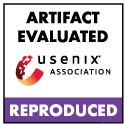