Bingyang Wu, Ruidong Zhu, and Zili Zhang, School of Computer Science, Peking University; Peng Sun, Shanghai AI Lab; Xuanzhe Liu and Xin Jin, School of Computer Science, Peking University
Low-rank adaptation (LoRA) is a popular approach to finetune pre-trained large language models (LLMs) to specific domains. This paper introduces dLoRA, an inference serving system for LoRA models. dLoRA achieves high serving efficiency by dynamically orchestrating requests and LoRA adapters in terms of two aspects: (i) dynamically merge and unmerge adapters with the base model; and (ii) dynamically migrate requests and adapters between different worker replicas. These capabilities are designed based on two insights. First, despite the allure of batching without merging a LoRA adapter into the base model, it is not always beneficial to unmerge, especially when the types of requests are skewed. Second, the autoregressive nature of LLM requests introduces load imbalance between worker replicas due to varying input and output lengths, even if the input requests are distributed uniformly to the replicas. We design a credit-based batching algorithm to decide when to merge and unmerge, and a request-adapter co-migration algorithm to decide when to migrate. The experimental results show that dLoRA improves the throughput by up to 57.9× and 26.0×, compared to vLLM and HugginFace PEFT, respectively. Compared to the concurrent work S-LoRA, dLoRA achieves up to 1.8× lower average latency.
OSDI '24 Open Access Sponsored by
King Abdullah University of Science and Technology (KAUST)
Open Access Media
USENIX is committed to Open Access to the research presented at our events. Papers and proceedings are freely available to everyone once the event begins. Any video, audio, and/or slides that are posted after the event are also free and open to everyone. Support USENIX and our commitment to Open Access.
author = {Bingyang Wu and Ruidong Zhu and Zili Zhang and Peng Sun and Xuanzhe Liu and Xin Jin},
title = {{dLoRA}: Dynamically Orchestrating Requests and Adapters for {LoRA} {LLM} Serving},
booktitle = {18th USENIX Symposium on Operating Systems Design and Implementation (OSDI 24)},
year = {2024},
isbn = {978-1-939133-40-3},
address = {Santa Clara, CA},
pages = {911--927},
url = {https://www.usenix.org/conference/osdi24/presentation/wu-bingyang},
publisher = {USENIX Association},
month = jul
}
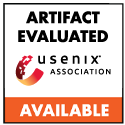
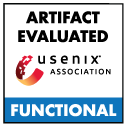
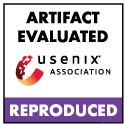