Daria Barteneva, Microsoft Azure
Machine Learning (ML) is becoming a part of many aspects of SRE life. As an SRE, we are (or will be soon) dealing with the challenge of serving ML models as part of a large distributed production system. Unfortunately the domain expertise required to build ML doesn't overlap with the expertise required to run large distributed system. The SRE community lacks standard practices and experiences that would allow us to operationalize ML and help to answer critical question: how exactly do we operate ML at scale reliably?
In this talk we will explore the (lack of) overlap between ML and SRE domains and discuss how we can help practitioners to solve common challenges. Scoping this talk to ML Observability we will be decomposing a complex system into its primary components helping engineers to bridge domain expertise gap in making ML systems more observable.
But when our production system serves ML models, relying only on traditional observability practices is not enough. We will review the characteristics and requirements specific to serving ML in production and discuss mechanisms that will help us to understand the end to end system reliability and quality.
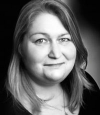
Daria is a Principal Site Reliability Engineer in Observability Engineering in Azure. With a background in Applied Mathematics, Artificial Intelligence, and Music, Daria is passionate about machine learning, diversity in tech, and opera. In her current role, Daria is focused on changing organisational culture, processes, and platforms to improve service reliability and on-call experience. She has spoken at conferences on various aspects of reliability and human factors that play a key role in engineering practices, and has written for O'Reilly. Daria is originally from Moscow, Russia, having spent 20 years in Portugal, 10 years in Ireland, and now lives in the Pacific NorthWest.

author = {Daria Barteneva},
title = {An {SRE} Approach to Monitoring {ML} in Production},
year = {2025},
address = {Santa Clara, CA},
publisher = {USENIX Association},
month = mar
}