Ian Neidel, Netflix
Content Delivery Network SREs are accustomed to metrics such as latency, bitrate, and dropped packets that measure how well we deliver content. However, as our team at Netflix expanded into ensuring good quality of experience for cloud gaming, a new challenge emerged: we must also be sure that what we deliver is fine as well. That is, we need to be able to automatically detect broken gameplay sessions and game breaking issues in a scalable way.
With a growing number of sessions and reams of logs per day, we turn to statistics and machine learning techniques to solve these otherwise difficult tasks at scale. In this talk we will cover the variety of metrics we use to infer brokenness, explain accessible methods to vectorize and cluster exception messages, and provide some insight into the statistics we use to find broken sessions, identify game breaking issues, and infer their impact with confidence.
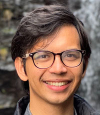
Ian Neidel is a SRE for Open Connect, Netflix’s in-house CDN. He works on Quality of Experience for Cloud Games, improving resiliency and realtime observability for Live Streaming, and automatic diagnosis and remediation of issues across Netflix’s distributed fleet of servers using Temporal — to choose a few. He attempts to back everything he and his software does in data where possible. Ian previously worked for two NASA centers and Amazon while an undergraduate studying Computer Science and Global Affairs at Yale.

author = {Ian Neidel},
title = {Using Statistical Techniques to Automatically Detect {Game-Breaking} Issues},
year = {2025},
address = {Santa Clara, CA},
publisher = {USENIX Association},
month = mar
}