Anamaya Sullerey and Brian Hansen, Meta
We share how we have transformed the way Monetization at Meta approaches machine learning training infrastructure management to unleash Efficiency and unlock Innovation. As AI model sizes and deployment footprints continue to explode, inefficient resource allocation and utilization are no longer just a nuisance – they're a major roadblock to innovation.
We'll dive into the cutting-edge strategies and real-world examples of how to use governance to:
- Drive ROI: Accurately measure and attribute the cost of ML training to focus on high ROI investments.
- Unlock hidden capacity: Maximize your existing resources and reduce waste
- Accelerate time-to-market: Streamline your ML development process and get to production faster
Through a case study of a successful ML training workload governance system, we'll explore the complexities of attributing costs in ML training to projects and share hard-won lessons from bridging the gap between research and production.
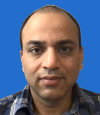
Anamaya Sullerey is a technical leader in the AdsML Production Engineering team, focused on capacity, efficiency, and reliability in the ML production environment. He has over two decades of broad experience across ML, software, compute and network systems, and silicon. Anamaya holds an MS in EE from Stanford University and a BTech in EE from IIT Kanpur.
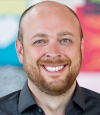
Brian leads the AdsML Production Engineering teams for Meta, focused on scaling machine learning in production environments. He has been a successful serial entrepreneur for two decades taking multiple start-ups from early to late stage growth. Throughout his career Brian has been a leader building global teams leveraging infrastructure to improve business performance.

author = {Anamaya Sullerey and Brian Hansen},
title = {Optimizing Machine Learning Training Infrastructure: A Governance Approach},
year = {2025},
address = {Santa Clara, CA},
publisher = {USENIX Association},
month = mar
}