Mazal Bethany, Andrew Seong, Samuel Henrique Silva, Nicole Beebe, Nishant Vishwamitra, and Peyman Najafirad, The University of Texas at San Antonio
Online Social Networks (OSNs) are increasingly used by perpetrators to harass their targets via the exchange of unsafe images. Furthermore, perpetrators have resorted to using advanced techniques like adversarial attacks to evade the detection of such images. To defend against this threat, OSNs use AI/ML-based detectors to flag unsafe images. However, these detectors cannot explain the regions of unsafe content for the obfuscation and inspection of such regions, and are also critically vulnerable to adversarial attacks that fool their detection. In this work, we first conduct an in-depth investigation into state-of-the-art explanation techniques and commercially-available unsafe image detectors and find that they are severely deficient against adversarial unsafe images. To address these deficiencies we design a new system that performs targeted obfuscation of unsafe adversarial images on social media using reconstruction to remove adversarial perturbations and counterfactual super region attribution explainability to explain unsafe image segments, and created a prototype called ProjectName. We demonstrate the effectiveness of our system with a large-scale evaluation on three common unsafe images: Sexually Explicit, Cyberbullying, and Self-Harm. Our evaluations of ProjectName on more than 64,000 real-world unsafe OSN images, and unsafe images found in the wild such as sexually explicit celebrity deepfakes and self-harm images show that it significantly neutralizes the threat of adversarial unsafe images, by safely obfuscating 91.47% of such images.
Open Access Media
USENIX is committed to Open Access to the research presented at our events. Papers and proceedings are freely available to everyone once the event begins. Any video, audio, and/or slides that are posted after the event are also free and open to everyone. Support USENIX and our commitment to Open Access.

author = {Mazal Bethany and Andrew Seong and Samuel Henrique Silva and Nicole Beebe and Nishant Vishwamitra and Peyman Najafirad},
title = {Towards Targeted Obfuscation of Adversarial Unsafe Images using Reconstruction and Counterfactual Super Region Attribution Explainability},
booktitle = {32nd USENIX Security Symposium (USENIX Security 23)},
year = {2023},
isbn = {978-1-939133-37-3},
address = {Anaheim, CA},
pages = {643--660},
url = {https://www.usenix.org/conference/usenixsecurity23/presentation/bethany},
publisher = {USENIX Association},
month = aug
}
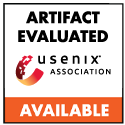