Kevin Eykholt, Taesung Lee, Douglas Schales, Jiyong Jang, and Ian Molloy, IBM Research; Masha Zorin, University of Cambridge
Machine learning models are known to be vulnerable to adversarial evasion attacks as illustrated by image classification models. Thoroughly understanding such attacks is critical in order to ensure the safety and robustness of critical AI tasks. However, most evasion attacks are difficult to deploy against a majority of AI systems because they have focused on image domain with only few constraints. An image is composed of homogeneous, numerical, continuous, and independent features, unlike many other input types to AI systems used in practice. Furthermore, some input types include additional semantic and functional constraints that must be observed to generate realistic adversarial inputs. In this work, we propose a new framework to enable the generation of adversarial inputs irrespective of the input type and task domain. Given an input and a set of pre-defined input transformations, our framework discovers a sequence of transformations that result in a semantically correct and functional adversarial input. We demonstrate the generality of our approach on several diverse machine learning tasks with various input representations. We also show the importance of generating adversarial examples as they enable the deployment of mitigation techniques.
Open Access Media
USENIX is committed to Open Access to the research presented at our events. Papers and proceedings are freely available to everyone once the event begins. Any video, audio, and/or slides that are posted after the event are also free and open to everyone. Support USENIX and our commitment to Open Access.
author = {Kevin Eykholt and Taesung Lee and Douglas Schales and Jiyong Jang and Ian Molloy},
title = {{URET}: Universal Robustness Evaluation Toolkit (for Evasion)},
booktitle = {32nd USENIX Security Symposium (USENIX Security 23)},
year = {2023},
isbn = {978-1-939133-37-3},
address = {Anaheim, CA},
pages = {3817--3833},
url = {https://www.usenix.org/conference/usenixsecurity23/presentation/eykholt},
publisher = {USENIX Association},
month = aug
}
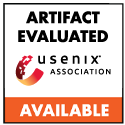
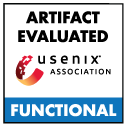
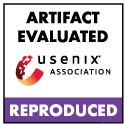