Yuanyuan Yuan, The Hong Kong University of Science and Technology and ETH Zurich; Shuai Wang, The Hong Kong University of Science and Technology; Zhendong Su, ETH Zurich
The objective of neural network (NN) robustness certification is to determine if a NN changes its predictions when mutations are made to its inputs. While most certification research studies pixel-level or a few geometrical-level and blurring operations over images, this paper proposes a novel framework, GCERT, which certifies NN robustness under a precise and unified form of diverse semantic-level image mutations. We formulate a comprehensive set of semantic-level image mutations uniformly as certain directions in the latent space of generative models. We identify two key properties, independence and continuity, that convert the latent space into a precise and analysis-friendly input space representation for certification. GCERT can be smoothly integrated with de facto complete, incomplete, or quantitative certification frameworks. With its precise input space representation, GCERT enables for the first time complete NN robustness certification with moderate cost under diverse semantic-level input mutations, such as weather-filter, style transfer, and perceptual changes (e.g., opening/closing eyes). We show that GCERT enables certifying NN robustness under various common and security-sensitive scenarios like autonomous driving.
Open Access Media
USENIX is committed to Open Access to the research presented at our events. Papers and proceedings are freely available to everyone once the event begins. Any video, audio, and/or slides that are posted after the event are also free and open to everyone. Support USENIX and our commitment to Open Access.

author = {Yuanyuan Yuan and Shuai Wang and Zhendong Su},
title = {Precise and Generalized Robustness Certification for Neural Networks},
booktitle = {32nd USENIX Security Symposium (USENIX Security 23)},
year = {2023},
isbn = {978-1-939133-37-3},
address = {Anaheim, CA},
pages = {4769--4786},
url = {https://www.usenix.org/conference/usenixsecurity23/presentation/yuan-yuanyuan-certification},
publisher = {USENIX Association},
month = aug
}
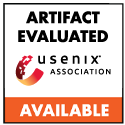