Zirui Huang, Yunlong Mao, and Sheng Zhong, Nanjing University
Machine-Learning-as-a-Service (MLaaS) is an emerging product to meet the market demand. However, end users are required to upload data to the remote server when using MLaaS, raising privacy concerns. Since the right to be forgotten came into effect, data unlearning has been widely supported in on-cloud products for removing users' private data from remote datasets and machine learning models. Plenty of machine unlearning methods have been proposed recently to erase the influence of forgotten data. Unfortunately, we find that machine unlearning makes the on-cloud model highly vulnerable to backdoor attacks. In this paper, we report a new threat against models with unlearning enabled and implement an Unlearning Activated Backdoor Attack with Influence-driven camouflage (UBA-Inf). Unlike conventional backdoor attacks, UBA-Inf provides a new backdoor approach for effectiveness and stealthiness by activating the camouflaged backdoor through machine unlearning. The proposed approach can be implemented using off-the-shelf backdoor generating algorithms. Moreover, UBA-Inf is an "on-demand" attack, offering fine-grained control of backdoor activation through unlearning requests, overcoming backdoor vanishing and exposure problems. By extensively evaluating UBA-Inf, we conclude that UBA-Inf is a powerful backdoor approach that improves stealthiness, robustness, and persistence.
Open Access Media
USENIX is committed to Open Access to the research presented at our events. Papers and proceedings are freely available to everyone once the event begins. Any video, audio, and/or slides that are posted after the event are also free and open to everyone. Support USENIX and our commitment to Open Access.

author = {Zirui Huang and Yunlong Mao and Sheng Zhong},
title = {{UBA-Inf}: Unlearning Activated Backdoor Attack with {Influence-Driven} Camouflage},
booktitle = {33rd USENIX Security Symposium (USENIX Security 24)},
year = {2024},
isbn = {978-1-939133-44-1},
address = {Philadelphia, PA},
pages = {4211--4228},
url = {https://www.usenix.org/conference/usenixsecurity24/presentation/huang-zirui},
publisher = {USENIX Association},
month = aug
}
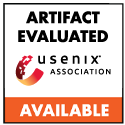
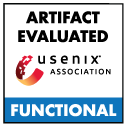
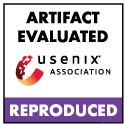