Seth Layton, Tyler Tucker, Daniel Olszewski, Kevin Warren, Kevin Butler, and Patrick Traynor, University of Florida
Deepfake media represents an important and growing threat not only to computing systems but to society at large. Datasets of image, video, and voice deepfakes are being created to assist researchers in building strong defenses against these emerging threats. However, despite the growing number of datasets and the relative diversity of their samples, little guidance exists to help researchers select datasets and then meaningfully contrast their results against prior efforts. To assist in this process, this paper presents the first systematization of deepfake media. Using traditional anomaly detection datasets as a baseline, we characterize the metrics, generation techniques, and class distributions of existing datasets. Through this process, we discover significant problems impacting the comparability of systems using these datasets, including unaccounted-for heavy class imbalance and reliance upon limited metrics. These observations have a potentially profound impact should such systems be transitioned to practice - as an example, we demonstrate that the widely-viewed best detector applied to a typical call center scenario would result in only 1 out of 333 flagged results being a true positive. To improve reproducibility and future comparisons, we provide a template for reporting results in this space and advocate for the release of model score files such that a wider range of statistics can easily be found and/or calculated. Through this, and our recommendations for improving dataset construction, we provide important steps to move this community forward.
Open Access Media
USENIX is committed to Open Access to the research presented at our events. Papers and proceedings are freely available to everyone once the event begins. Any video, audio, and/or slides that are posted after the event are also free and open to everyone. Support USENIX and our commitment to Open Access.
author = {Seth Layton and Tyler Tucker and Daniel Olszewski and Kevin Warren and Kevin Butler and Patrick Traynor},
title = {{SoK}: The Good, The Bad, and The Unbalanced: Measuring Structural Limitations of Deepfake Media Datasets},
booktitle = {33rd USENIX Security Symposium (USENIX Security 24)},
year = {2024},
isbn = {978-1-939133-44-1},
address = {Philadelphia, PA},
pages = {1027--1044},
url = {https://www.usenix.org/conference/usenixsecurity24/presentation/layton},
publisher = {USENIX Association},
month = aug
}
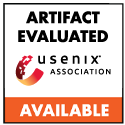
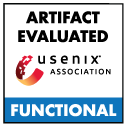
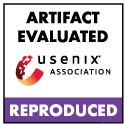