Robocalls – automated spam calls – have frustrated phone users for well over a decade [1]. In the United States, the never-ending flow of robocalls has forced people to stop answering most calls. On rare occasions, when someone does answer a call from an unknown number, it is usually a robocall urging them to sign-up for an auto warranty scheme or threatening to “suspend” their social security number.
While many now ignore labeled or unlabeled nuisance calls, they are still effective. Some of the most effective robocalls specifically target more vulnerable populations, including seniors, recent immigrants, and non-English speakers residing in the US. The FTC estimates that victims of phone-originated scams have lost over $194 Million in the first three months of 2023 alone [2].
The main approach to stopping fraudulent or other illegal robocalls is to identify the source of unlawful robocalls and disconnect them from the phone network. In the US, regulatory agencies (the FCC and the FTC), State Attorneys General, and law enforcement agencies are responsible for taking action against illegal robocalling operations. These stakeholders collect robocall audio recordings from commercially operated honeypots, from the public, and other sources. However, they do not have automated tools to analyze vast amounts of robocall data and uncover investigative leads in a timely manner.
In our recently published paper at USENIX Security 2023, we develop SnorCall [3] – a robocall audio content analysis pipeline that enables robocall domain experts to swiftly analyze vast amounts of robocall audio data. SnorCall builds on our prior work published at USENIX Security 2020 [4], which takes raw audio and call metadata collected from honeypots and identifies “campaigns” of different calls with nearly identical content. SnorCall extends the prior work to produce transcripts from each campaign and extract content labels and other pertinent information about each campaign based on the content of the call. We demonstrate SnorCall’s capabilities by extracting insights from more than a million robocalls collected in our honeypot over a 23-month period.
Robocalling operations uniformly want a call recipient to take some action, like continuing to a live human agent or purchasing a product. Using state-of-the-art natural language processing (NLP) techniques, we analyzed over 232k robocalls to extract and analyze robocall calls-to-action. For example, political robocalls may encourage people to vote, whereas social security scam calls may threaten people to hastily call a specific phone number to avoid “an arrest warrant.”
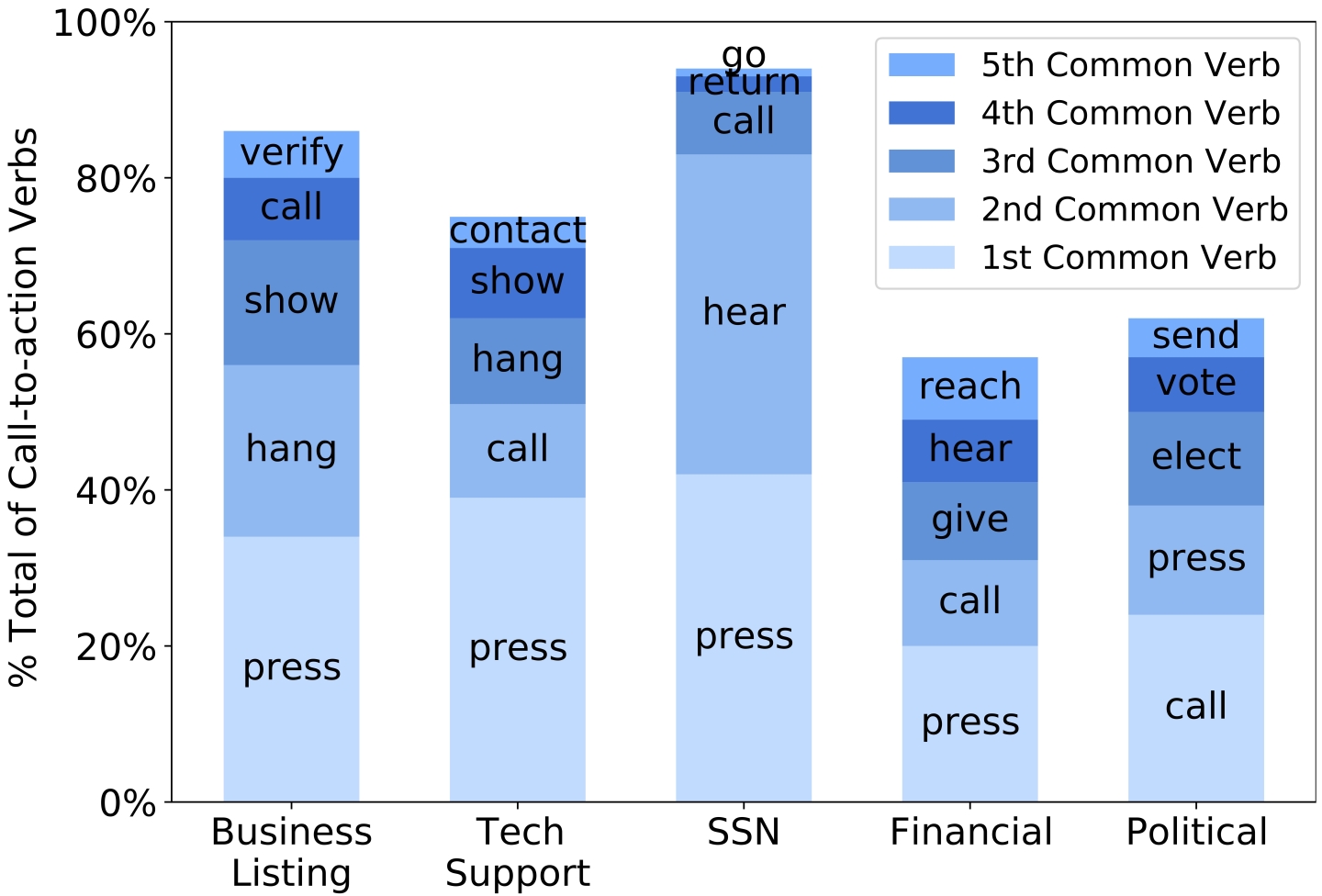
SnorCall enables investigators to extract forensic leads in the form of callback numbers that can aid further investigation into the robocalling infrastructure and the identity of the robocall originators. Nearly 50% of all robocalls used a callback number to engage with their target. SnorCall accurately identified over 3,000 unique callback numbers from call audio and found that some callback numbers are shared across multiple campaigns, indicating shared infrastructure across multiple campaigns. Robocalls often spoof the caller ID when generating phone calls to obscure their identity and improve the likelihood of an answer. We found that more than 95% of robocalls had a different caller ID and callback number. While the Caller ID can be anything, it is in the interest of the robocaller to provide a real, active callback number so that when recipients call back, they can speak with a live representative. Since these numbers lead back to the robocalling infrastructure, investigators can use ownership information of callback numbers to track down the identity of illegal robocallers and pursue legal action against them.
"From US Drug Enforcement Administration the reason of this call [sic] to suspend your social insurance number on an immediate basis as we have received suspicious Trails of information with your social security number the moment you receive this message. You need to get back to us to avoid legal consequences on XXX-XXX-XXXX. I repeat XXX-XXX-XXXX. Thank you."
Regulators and investigators responsible for shutting down illegal robocalling operations are overwhelmed by the sheer volume of unlawful robocall activity in the US. The absence of automated tools to analyze robocall audio data has forced them to rely on manual analysis to uncover instances of illegal intent from vast amounts of robocall recordings. We developed SnorCall to address this problem.
The flexibility and robust design of SnorCall empowers robocall domain experts to sift through vast amounts of robocall audio data and selectively uncover robocall categories of interest.
We demonstrate SnorCall’s ability to identify specific types of robocalls by developing high-accuracy labelers for five robocall categories. These labelers helped us measure the relative prevalence of various robocall types, as shown in the table below. By narrowing the focus of our analysis to specific categories, we could study the operational characteristics of Social Social Security scams, track the evolution of Tech Support scams, and explore how robocalls take advantage of societal events to defraud their victims.
Category | Social Security Scams | Tech Support Scams | Political Robocalls | Financial Robocalls | Business Listing Robocalls |
---|---|---|---|---|---|
Number of Calls (% of English calls) | 8,292 (3.66%) | 8,402 (3.71%) | 11,727 (5.18%) | 57,839 (25.54%) | 24,316 (10.74%) |
Number of Campaigns (% of English campaigns) | 1,304 (5.17%) | 2,696 (10.70%) | 1,226 (4.86%) | 4,638 (18.40%) | 1,260 (4.99%) |
This capability of SnorCall is crucial for investigators and regulators to focus their investigative efforts on illegal robocalls (e.g., Social Security scams, Tech Support scams, etc.) while discarding nuisance telemarketing or other benign robocalls like public safety notices.
A well-known Social Security scam involves an impersonator threatening their victims while persuading them to reveal sensitive information (like their SSN) or extort money using a false sense of authority. We uncovered over 700 campaigns containing about 5,000 such calls.
“This call is regarding to [sic] your social security number. We found some fraudulent activities under your name and [an] arrest warrant has been issued and your Social Security would be suspended soon. Please press one to talk with [an] officer right away. I repeat, please press one to talk with [an] officer right away. Thank you.”
Interestingly, some Social Security scams have started adopting a non-intimidating tactic. Seeming well-intended, the caller claims to be a “Social Security Disability Advisor” and targets people eligible to seek Social Security benefits. In the US, Social Security disability benefits are sought by people who cannot work due to illness or injury.
"Hello, my name is Audrey and I’m a social security disability advisor with national disability on a recorded line. And my call back number is XXX-XXX-XXXX. Now I show here that you recently inquired about your eligibility for Social Security disability benefits. Can you hear me?[sic] Okay?”
Just like legitimate businesses, Social Security scam operations were disrupted during the onset of the COVID-19 pandemic. As many countries started imposing local restrictions and lockdowns in March 2020, there was a substantial decline in the number of Social Security scam calls. Since most lockdown restrictions prohibited people from commuting to work, it directly affected scammers who were operating from dedicated work locations with office-like infrastructure. This finding reinforces that robocalling scam operations are usually large and well-organized affairs.
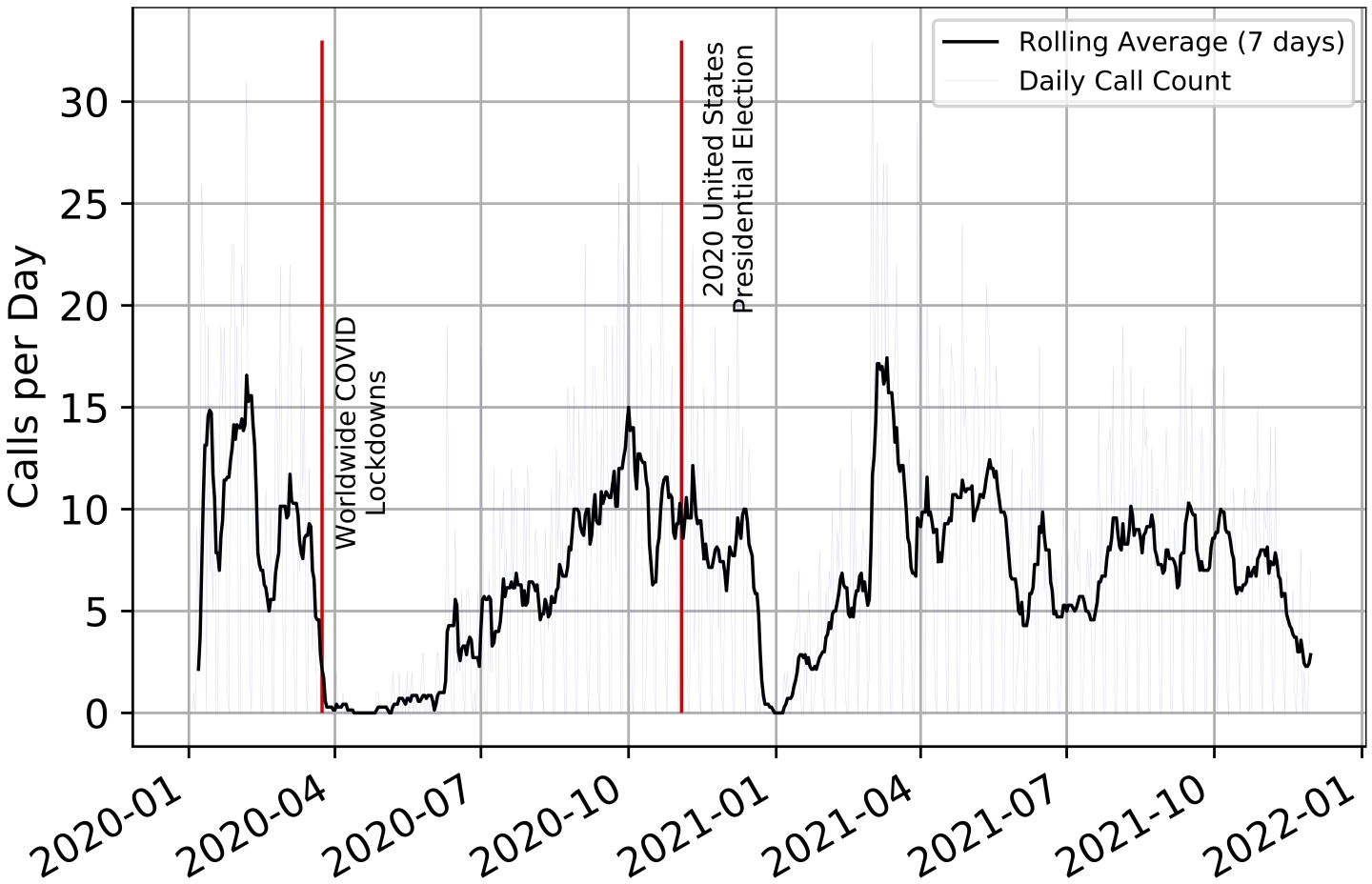
Tech support scammers impersonate well-known technology companies and businesses to extract payment from victims for fake repairs or services. However, Amazon impersonation scams are multiple orders of magnitude greater than well-known Windows or Microsoft tech support scams. These calls falsely claim to represent Amazon’s fraud department citing discrepancies in the target’s Amazon accounts. Other variants of Amazon tech support scams offer to remediate an erroneous Amazon Prime membership charge or refund an Apple product order (MacBook, AidPods, iPhone, etc). By studying the dollar amounts requested within each call, we estimate that the median tech support scam call attempts to defraud its victims of about $400.
“John from Amazon customer service. My employer ID is AMC, 2516, and I’m calling you about your Amazon Prime account. I wanted to inform you that your Amazon Prime account is being compromised, as long as an order for an iPhone 10 worth $549. For which the card attached to your Amazon account is being charged. We have placed, hold on it. As that order seems to be fraudulent. So please call us on this toll free number XXX-XXXX. I repeat one XXX-XXX-XXXX to talk to an Amazon fraud department executive. Thank you.”
“Purchase from Amazon shopping. This call is to inform you that your purchase for Apple MacBook Pro and Apple airpods will be delivered by tomorrow evening and $1,537.35 Home in debited from your account for this purchase. If you authorize this charge then no action required, and if you did not authorize this charge, then press one to connect to Amazon customer representative for cancellation charge.”
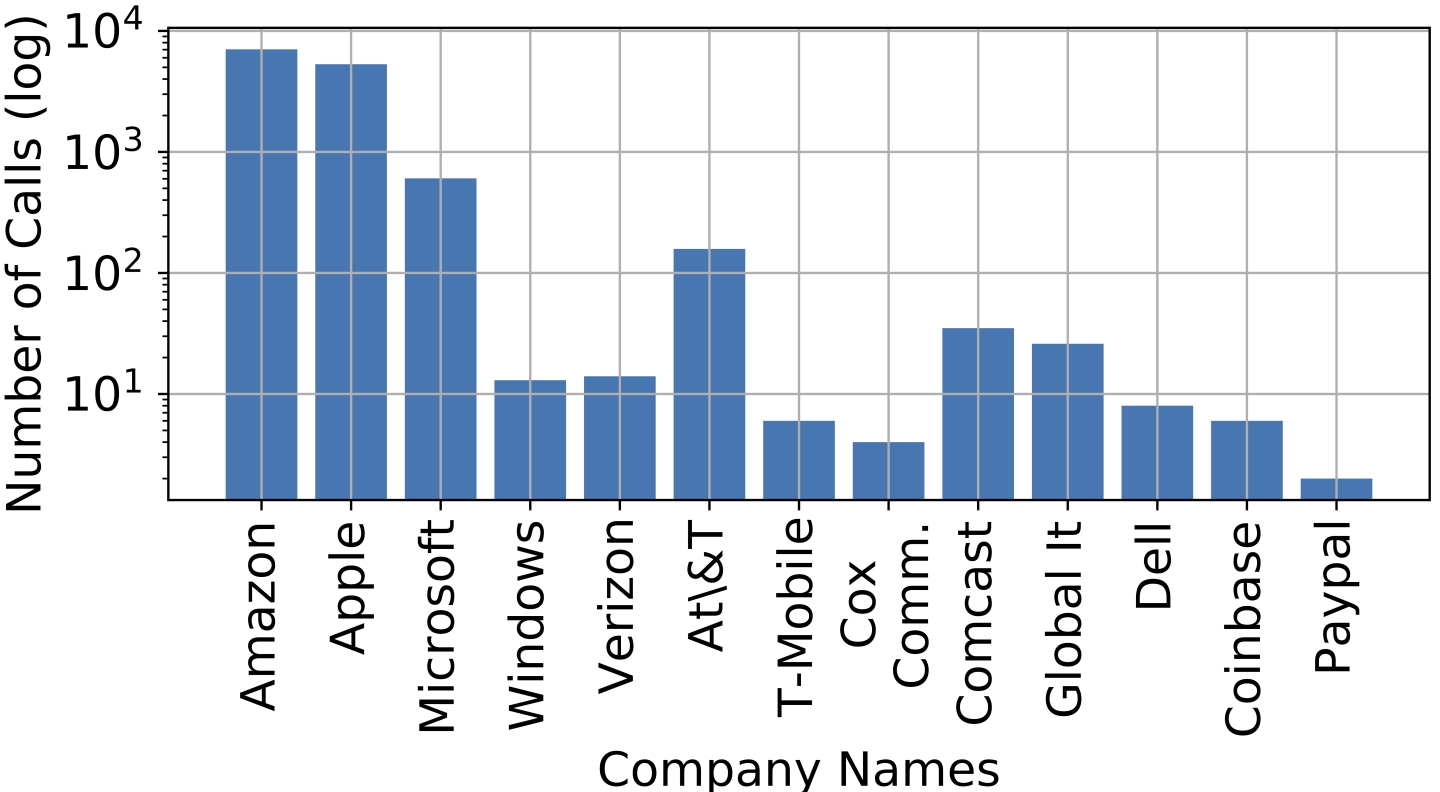
SnorCall also uncovered robocalls targeting cryptocurrency wallet users, where the caller impersonated Coinbase support agents and offered assistance to recover locked Bitcoin. By examining the call patterns of these campaigns on our honeypot, we determined that these cryptocurrency scam calls target specific phone numbers to achieve higher success rates. In contrast, Amazon and Windows tech support callers seem to target callers at random.
“Dear coinbase. Customer your coinbase account, temporarily disabled indicates that your account currently has a restriction, potentially related to a security concern. This restriction requires a coinbase Security review to be removed. This restriction, may be applied for several reasons. Our security team suspected that your account was being targeted by a malicious user. Please. Press one to contact customer support for recover, your Bitcoin, please press one for recover your Bitcoin.“
Illegal robocalling operations swiftly adapt to take advantage of major societal events to defraud their targets. SnorCall uncovered robocalling campaigns misrepresenting political events to cause financial harm or steal personal information. These campaigns were misrepresenting political current events and claimed to represent a non-existent “Economic Impact Student Loan Forgiveness Program recently put into effect by the Biden Administration.” While proposals for student loan forgiveness were being publicly discussed at the time, no such program was established during the robocall campaign’s activity. Given that the claimed program did not exist, it is unclear if victims will be offered loan products or if their personal information will simply be stolen. SnorCall labeled these previously-unseen campaigns as both “Political” and “Financial”, correctly capturinsg the nuances of the scam while highlighting a novel tactic from ever-evolving robocallers.
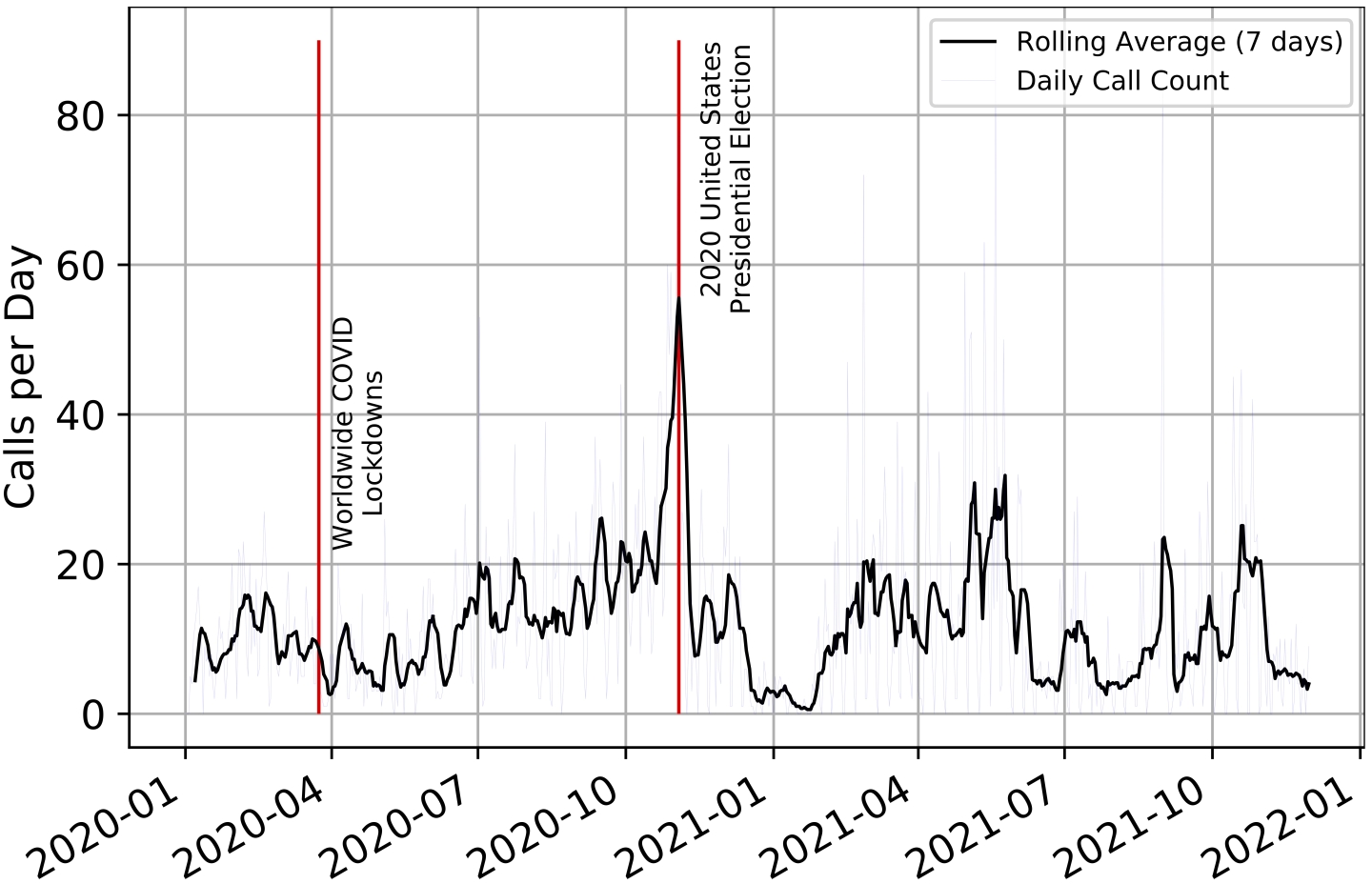
Robocalls continue to be one of the most visible and frustrating network security issues, and the vast scale of the problem is itself one of the factors preventing positive progress on the problem. Current approaches, from the FTC’s Do-Not-Call list to call origin signing with STIR/SHAKEN, have proven ineffective at enforcing good behavior and prosecuting perpetrators. The SnorCall pipeline offers a powerful complementary approach to understand, monitor, and collect evidence against abuse actors at the vast scale of the problem.
SnorCall shows how to quantify different scam and legitimate robocall topics, determine which organizations are referenced in these calls, estimate the average amounts solicited in scam calls, and identify actual infrastructure used in campaigns. Regulators, carriers, anti-robocall product vendors, and researchers can use SnorCall to obtain powerful and accurate analyses of robocall content and trends that can be used to inform the public and collect details that lead directly to stopping abuse actors.
This material is based upon work supported by the National Science Foundation under grant numbers CNS-1849994 and CNS-2142930. This work was partially supported by funds from the 2020 Facebook Internet Defense Prize. This material is based upon work supported by the Google Cloud Research Credits program with the award GCP19980904. We thank Bandwidth Inc. for their support and for providing VoIP service and phone numbers for our honeypot. Any opinions, findings, and conclusions or recommendations expressed in this material are those of the authors and do not necessarily reflect the views of the National Science Foundation, other funding agencies or financial supporters, or any other party.