Mengwei Xu, Dongqi Cai, Yaozong Wu, Xiang Li, and Shangguang Wang, Beijing University of Posts and Telecommunications (BUPT)
Large Language Models (LLMs) are transforming the landscape of mobile intelligence. Federated Learning (FL), a method to preserve user data privacy, is often employed in fine-tuning LLMs to downstream mobile tasks, i.e., FedLLM. A vital challenge of FedLLM is the tension between LLM complexity and resource constraint of mobile devices.
In response to this challenge, this work introduces FwdFL, an innovative FL protocol designed to enhance the FedLLM efficiency. The key idea of FwdFL is to employ backpropagation (BP)-free training methods, requiring devices only to execute ''perturbed inferences''. Consequently, FwdFL delivers way better memory efficiency and time efficiency (expedited by mobile NPUs and an expanded array of participant devices). FwdFL centers around three key designs: (1) it combines BP-free training with parameter-efficient training methods, an essential way to scale the approach to the LLM era; (2) it systematically and adaptively allocates computational loads across devices, striking a careful balance between convergence speed and accuracy; (3) it discriminatively samples perturbed predictions that are more valuable to model convergence. Comprehensive experiments illustrate FwdFL's significant advantages over conventional methods, including up to three orders of magnitude faster convergence and a 4.6× reduction in memory footprint. Uniquely, FwdFL paves the way for federated billion-parameter LLMs such as LLaMA on COTS mobile devices -- a feat previously unattained.
USENIX ATC '24 Open Access Sponsored by
King Abdullah University of Science and Technology (KAUST)
Open Access Media
USENIX is committed to Open Access to the research presented at our events. Papers and proceedings are freely available to everyone once the event begins. Any video, audio, and/or slides that are posted after the event are also free and open to everyone. Support USENIX and our commitment to Open Access.

author = {Mengwei Xu and Dongqi Cai and Yaozong Wu and Xiang Li and Shangguang Wang},
title = {{FwdLLM}: Efficient Federated Finetuning of Large Language Models with Perturbed Inferences},
booktitle = {2024 USENIX Annual Technical Conference (USENIX ATC 24)},
year = {2024},
isbn = {978-1-939133-41-0},
address = {Santa Clara, CA},
pages = {579--596},
url = {https://www.usenix.org/conference/atc24/presentation/xu-mengwei},
publisher = {USENIX Association},
month = jul
}
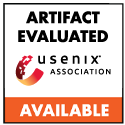
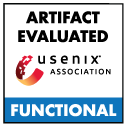
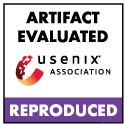